Let’s face it, a lot of a data engineer’s time is spent waiting to see if things executed as expected or for data to be refreshed; We write pipelines, buy expensive replication software, or sometime manually move files (I hope we still aren’t in this day and age), and in the end all of this has a cost associated with it when working in a cloud environment. In the case of Databricks jobs, we often find ourselves creating clusters just to move data, where the cluster lays dormant for the most part during these extractions. In my eyes, that’s wasteful and could probably be improved upon.
Continue reading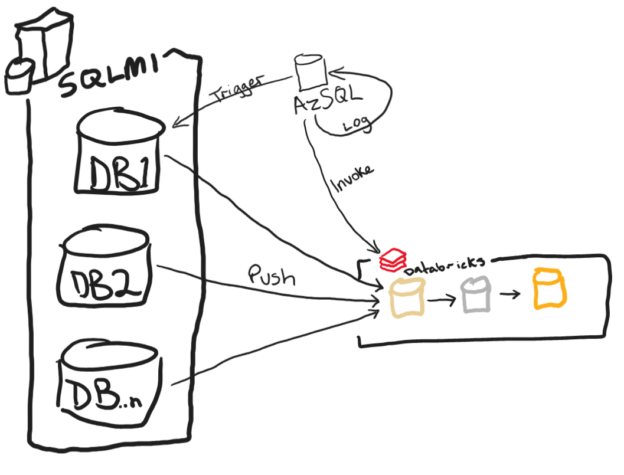